Practice makes perfect
AI could be the key to really exploiting the potential of digitalization. Endress+Hauser is working together with customers and partners in a step-by-step exploration of these new technologies – and in doing so is underlining their true added value.
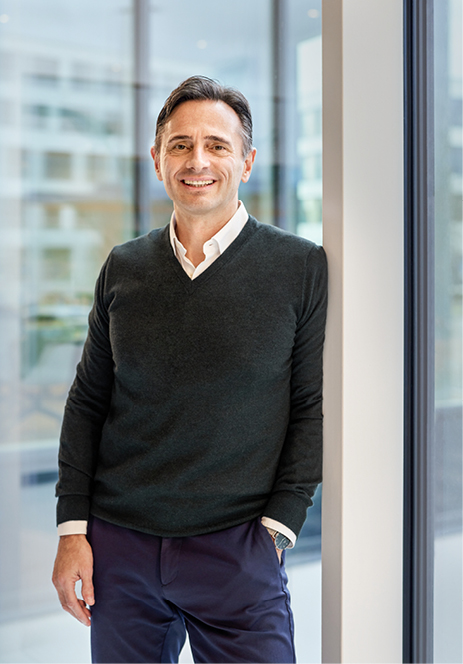
“AI is not a quick fix”
Artificial intelligence has been in commercial use for years. Now it’s set to boost efficiency in industrial plants. Digital strategist Marco Colucci explains where the potential of the new technology lies and how Endress+Hauser is helping customers prepare.
INNOVATION
There is considerable hype surrounding artificial intelligence these days. Some even tout it as a universal solution for all process-related problems. What is your take on the technology from the Endress+Hauser perspective?
For me, artificial intelligence is first and foremost a new driver of insights. In the digitalization context, AI combined with data acquired from our intelligent sensors installed in industrial plants should allow users to make better decisions based on the insights gained and thus unlock new opportunities for plant optimization. Chemical, food & beverage and life sciences count among industries that are highly automated already. Our customers there believe that intelligent solutions have the potential to improve efficiency by an average five percent, albeit only in certain areas. While there is a lot that AI can do, it’s in no way a panacea.
In what applications might AI deliver the greatest added value?
From developing numerous applications for customers over the years, I believe the greatest benefit lies in areas where AI can transcend the limits of physical measurement technology; here we’re talking predictive quality, predictive reliability and digital twins. The latter could use AI for autonomously adapting to changed system conditions. Predictive reliability is about reliability statements on the measurement performance of our instruments, as well as optimizing their calibration intervals. Statistical models are the present-day tool for analyzing historical calibration data; in future an algorithm could additionally incorporate monitoring data from our instruments equipped with Heartbeat Technology.
Predictive quality is frequently based on soft sensors able to determine parameters that today’s physical sensors are unable to monitor, or only in the lab or using the human senses. To accomplish that, we combine hardware sensors with an AI model and provide the new parameters inline. Soft sensors close what have until now been process information gaps. That’s why I view them as a highly important field for the future!
What about predictive maintenance? Many consider it a prime example of AI applications…
Predictive maintenance with AI is of course an important thing for the process industry. The idea is to improve plant availability through timely detection of impending component failures and scheduling specific maintenance activities accordingly. That being said, plant operators are not necessarily focused on our instruments so much as on wear-intensive mechanical and rotating components such as pumps and valves. Our sensor data could conceivably support predictive maintenance of these assets. Moreover, we are currently working on an AI solution for our instruments deployed in highly demanding applications where deposit formation, abrasion and corrosion are issues.
At Endress+Hauser and in the process industry in general, the use of AI has barely progressed beyond pilot projects. What makes transitioning to broad-based deployment difficult?
There are several reasons. For one thing, industry and manufacturers are still in an exploratory phase. Many attempts failed to yield hoped-for efficiency gains – or the solution is not scalable. Secondly, use cases cannot be developed overnight. Co-innovation stands front and center here; we must delve deep into the customer’s application know-how, get hold of the right data, then put it into the cloud, from where we can do the interpreting jointly with the customer. Aspects such as data access, interoperability, cybersecurity, the right mindset in the company and a whole lot more also have parts to play. AI is not a quick fix. And it’s not an issue that individual companies can drive forward all on their own.
So there is still work to be done before AI can become a success?
When planning new systems in particular, it is essential to think about digitalization and AI at the same time. We are currently involved in many water and wastewater projects where the measurement technology is selected with this in mind, and the plant design already contains provision for the requisite IT and OT infrastructure. Error-free, structured data is the first step, its management and visualization the second step, and the two together a prerequisite for the third step of algorithmic analysis.
Published 30.05.2024, last updated 18.06.2024.
Dive into the world of the process industry through new exciting stories every month with our «changes» newsletter!
Subscribe to newsletter