Getting more out of AI - together
Optimizing processes with AI requires users and providers to embark on a shared journey. Endress+Hauser follows a standardized procedure, ensuring transparency at every step and fostering trust.
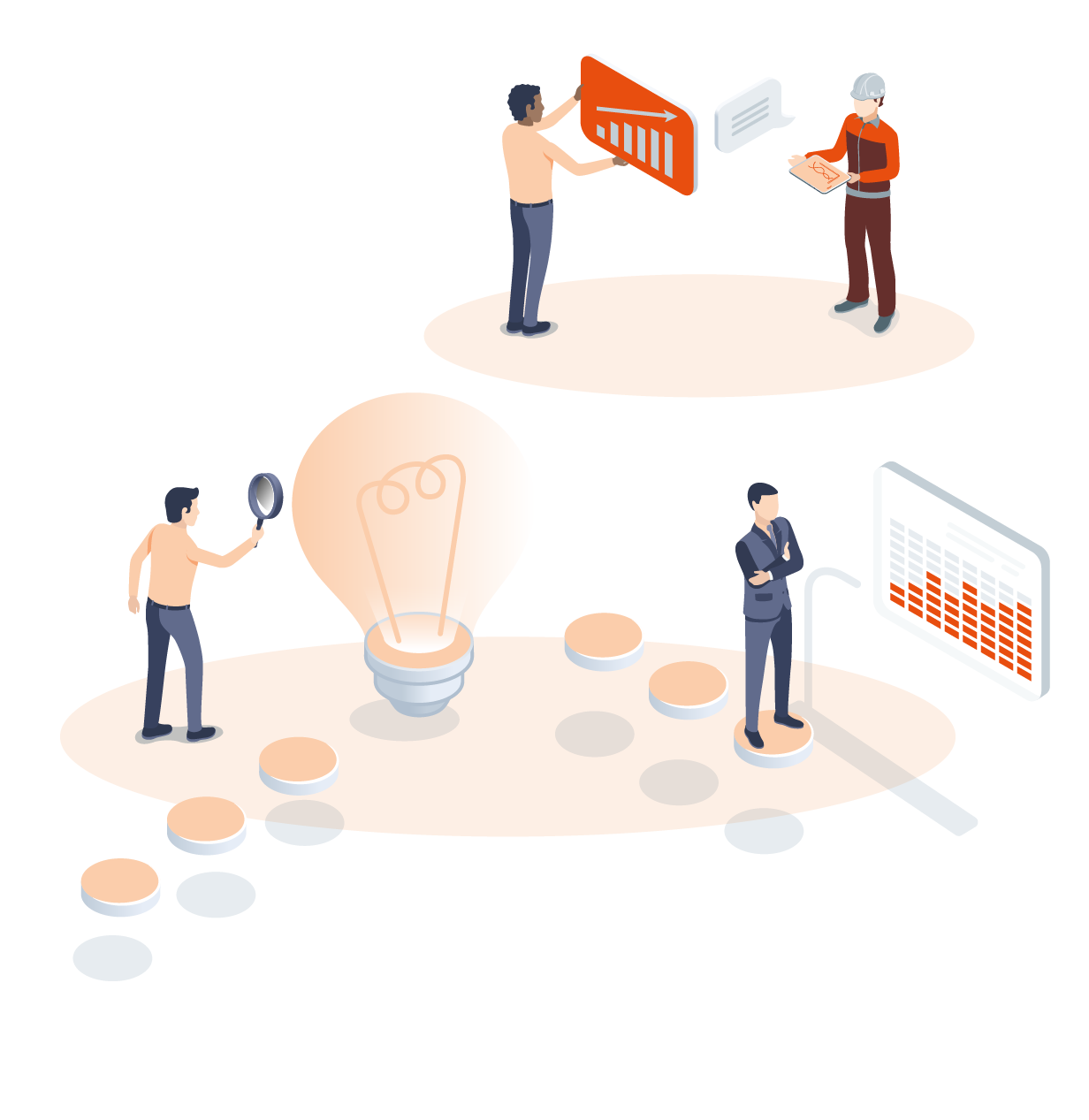
JOINING FORCES
Endress+Hauser believes in joint innovation with customers to develop AI solutions. The initiative for this may come from either side. “Sometimes it is our users who ask us things like whether we can get more out of an instrument by leveraging additional data and information,” says Dr Rebecca Page, a data scientist working in R&D at the flow measurement technology product center. With an end-to-end application involving all manner of measurement variables and instruments, Endress+Hauser will often be the one to approach the customer. “Our industry managers with their expertise and global network know exactly where their customers’ pain points are. In many cases, people have been thinking for quite some time about measurement-based solutions. But only now, with the digital transformation and AI, has it become possible to put this approach into action,” Page says.
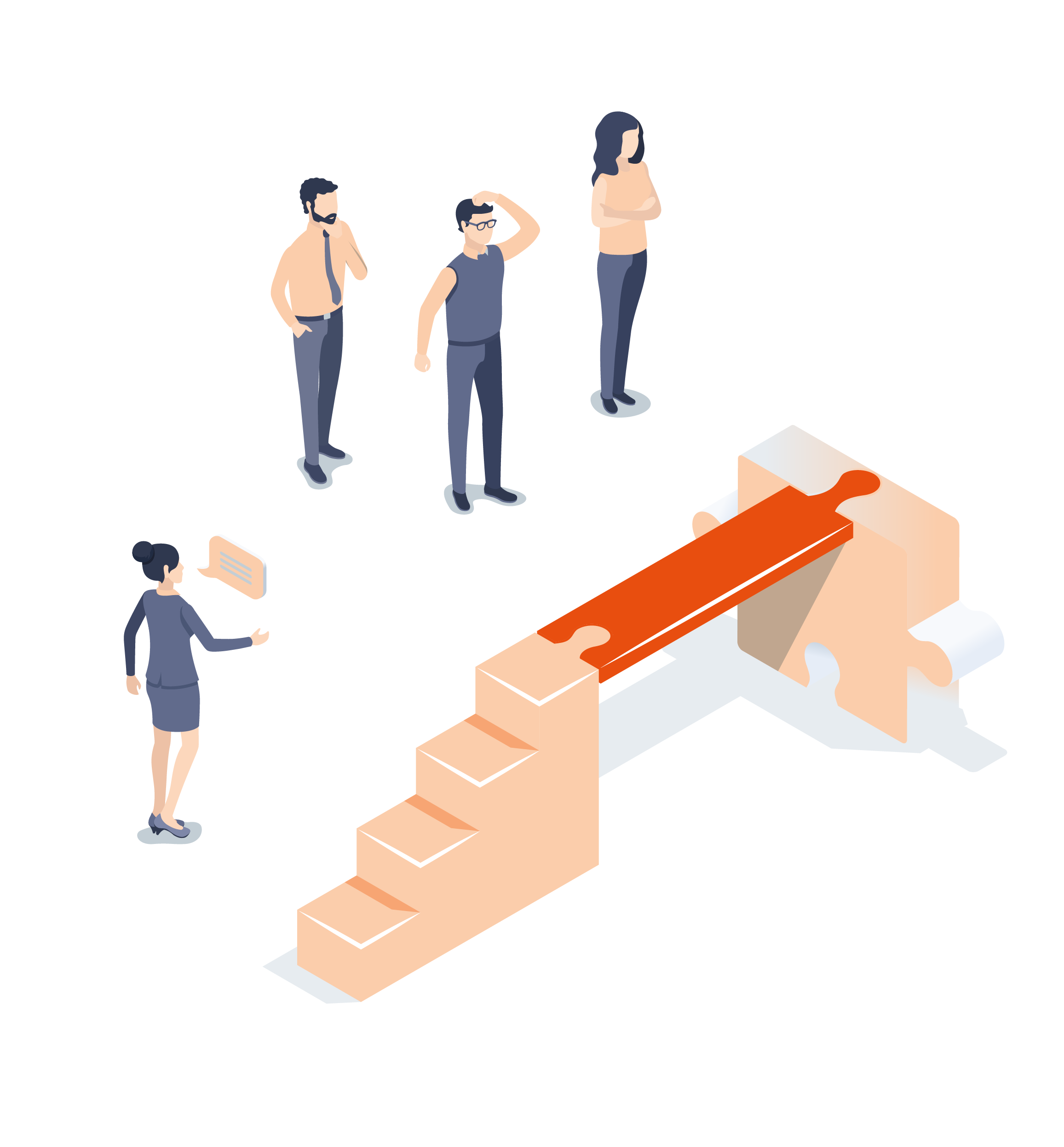
STARTING WITH A CLEAR GOAL
If everyone is keen to work together, Endress+Hauser and the customer will go through a standardized model for data mining projects (CRISP-DM). It all starts with understanding the business. For an end-to-end application this means asking various questions: What exactly does the process look like? What is the challenge? What improvements are users hoping to see? What does Endress+Hauser want to achieve with the project – and what resources and contributions does Endress+Hauser need for it to be a success? What do both partners bring to the table – and where do their skills and expertise lie? “Transparency is crucial here, along with clearly defining a common goal and the exact path for getting there,” Page notes. “Both sides have to trust each other, communicate well and want to learn – and users must be open to the idea of digitalization.”
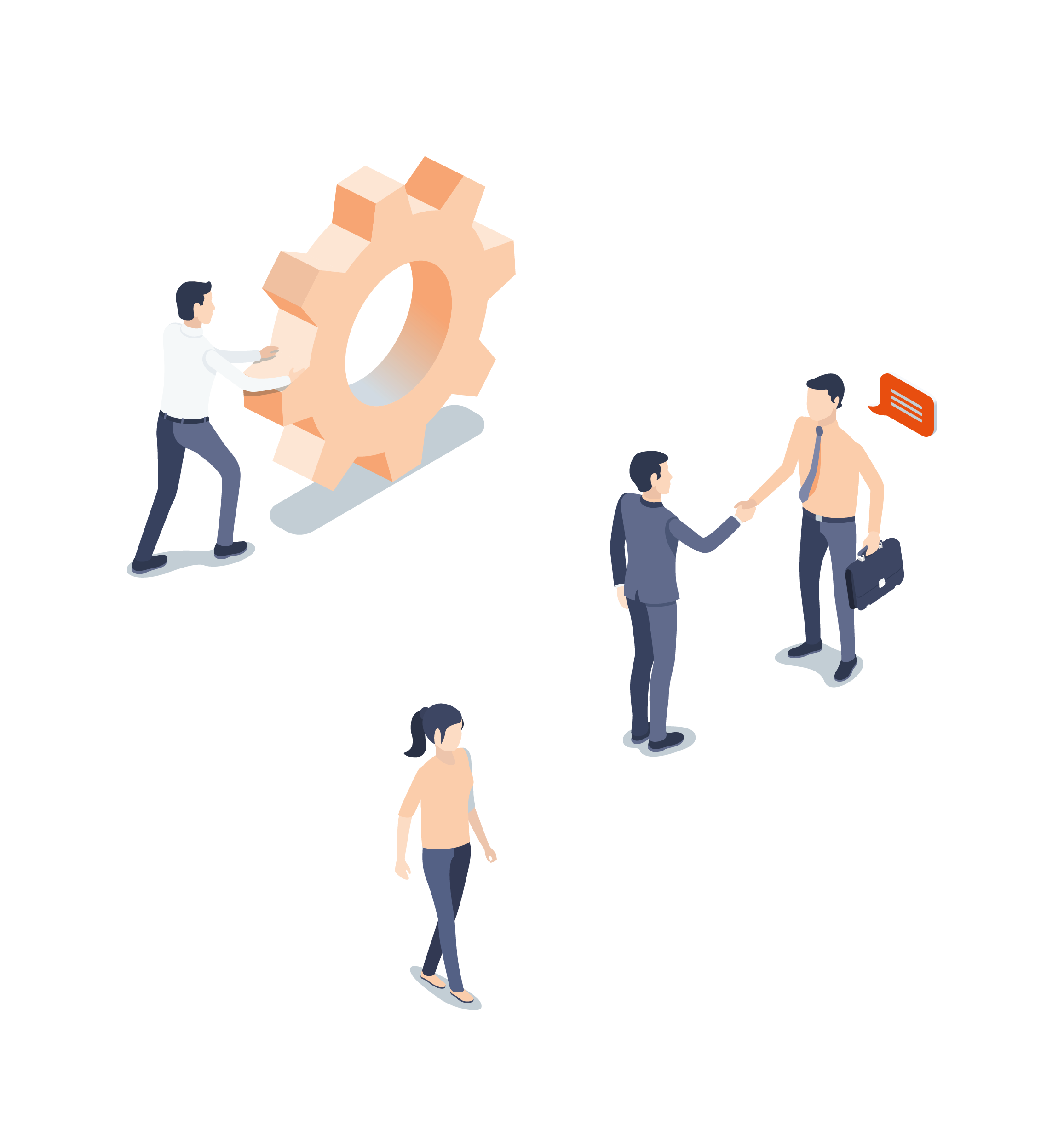
UNDERSTANDING THE PROCESS AND DATA
In the next phase, data scientists at Endress+Hauser receive measurement datasets to determine things such as the critical parameters and the control options that offer starting points to solve the problem. “Right now, we’re working on a predevelopment project with a customer in the mining industry to optimize a thickener,” Page explains. The process for separating solids from liquids is to be optimized with the help of AI. In addition, the layer of solid matter that settles on the bottom of the tank should have a certain density. “Another part of this is a deep dive on site with the process engineers,” Page says. “We need to gain profound insight into the process and then compare it against what we see in the data. The crucial point is to use the data to discern patterns that have an impact on the process.”
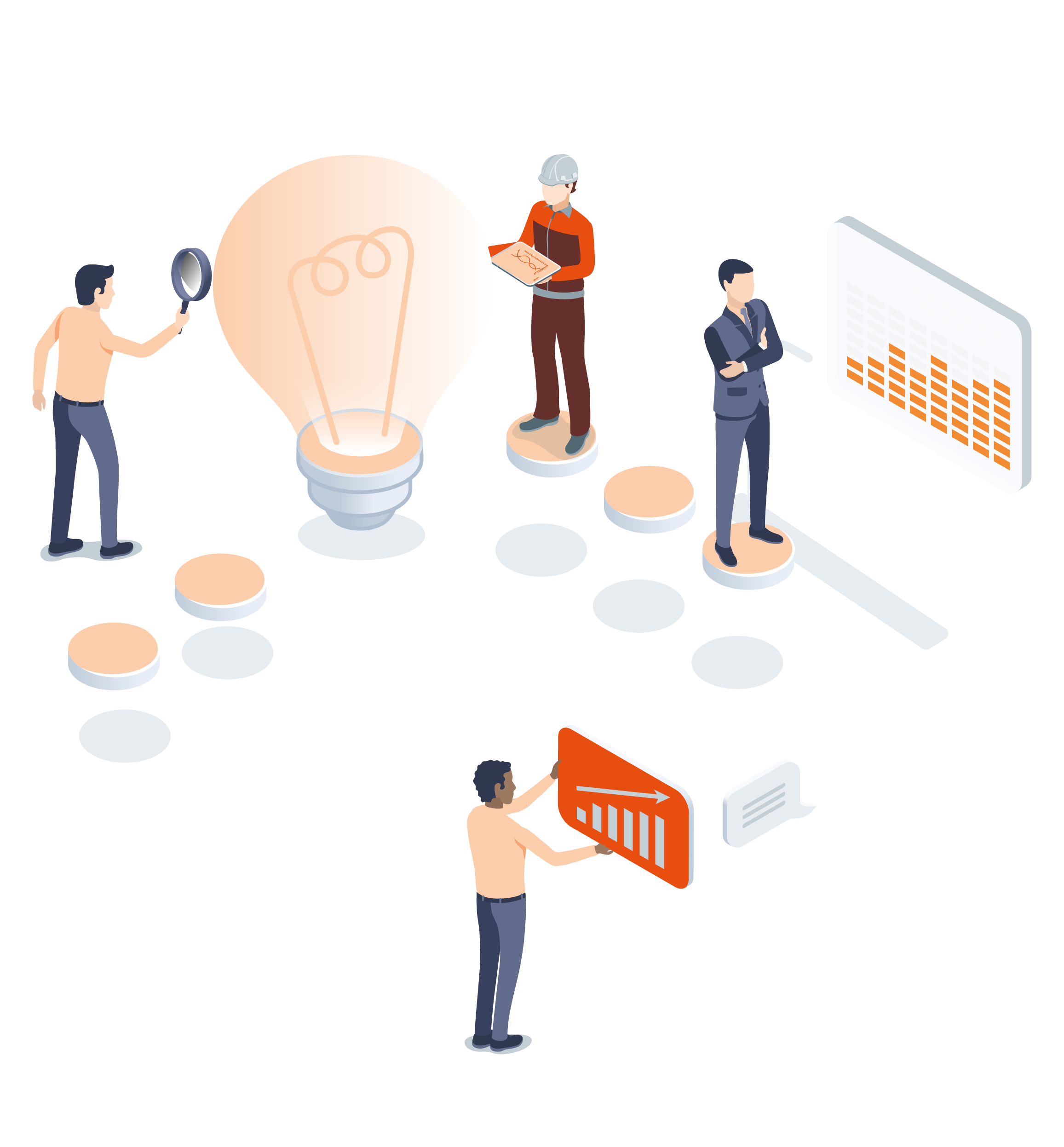
BRIDGING GAPS
If there are gaps in the data, for example because an interval between measurements is too long or key parameters are missing, Endress+Hauser steps in to bridge them. “Such gaps are often the consequence
of manual steps such as sampling,” Page explains. In the case of the thickener, the solid content of the overflow was going for lab analysis just once a day. “We’ve added various new measurements to record the dynamics of the process in between times when the samples go to the lab. That’s an immediate gain in transparency for users,” she says.
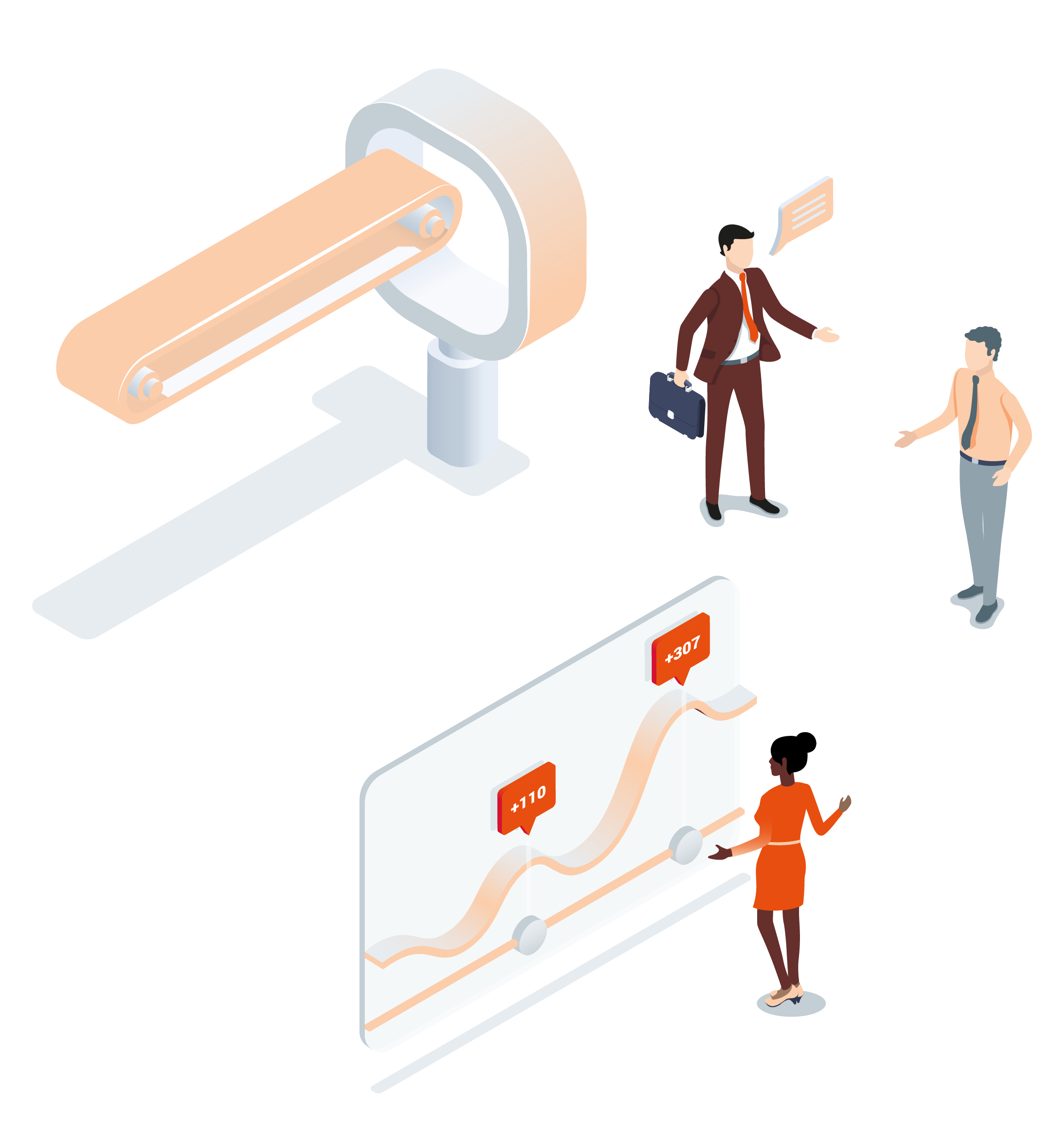
FINDING THE RIGHT MODEL
Data for the predevelopment project then flows via edge devices into the cloud. The result is even larger datasets for ongoing analysis and interpretation with help from the customer – looking for trends, variability and outliers. A final dataset forms the basis for developing a suitable AI model. “In applications where the many measuring devices in use make relationships more complex, machine learning can provide support,” Page explains. The model is tested extensively, first with simulated measurements and then real ones. “This is where we work with the customer to see whether the model fits, reflects reality and offers the added value they are seeking, or whether we need to change something.” After that, the model is configured, implemented in the customer’s environment – with or without a cloud connection, as the customer prefers – and monitored further.
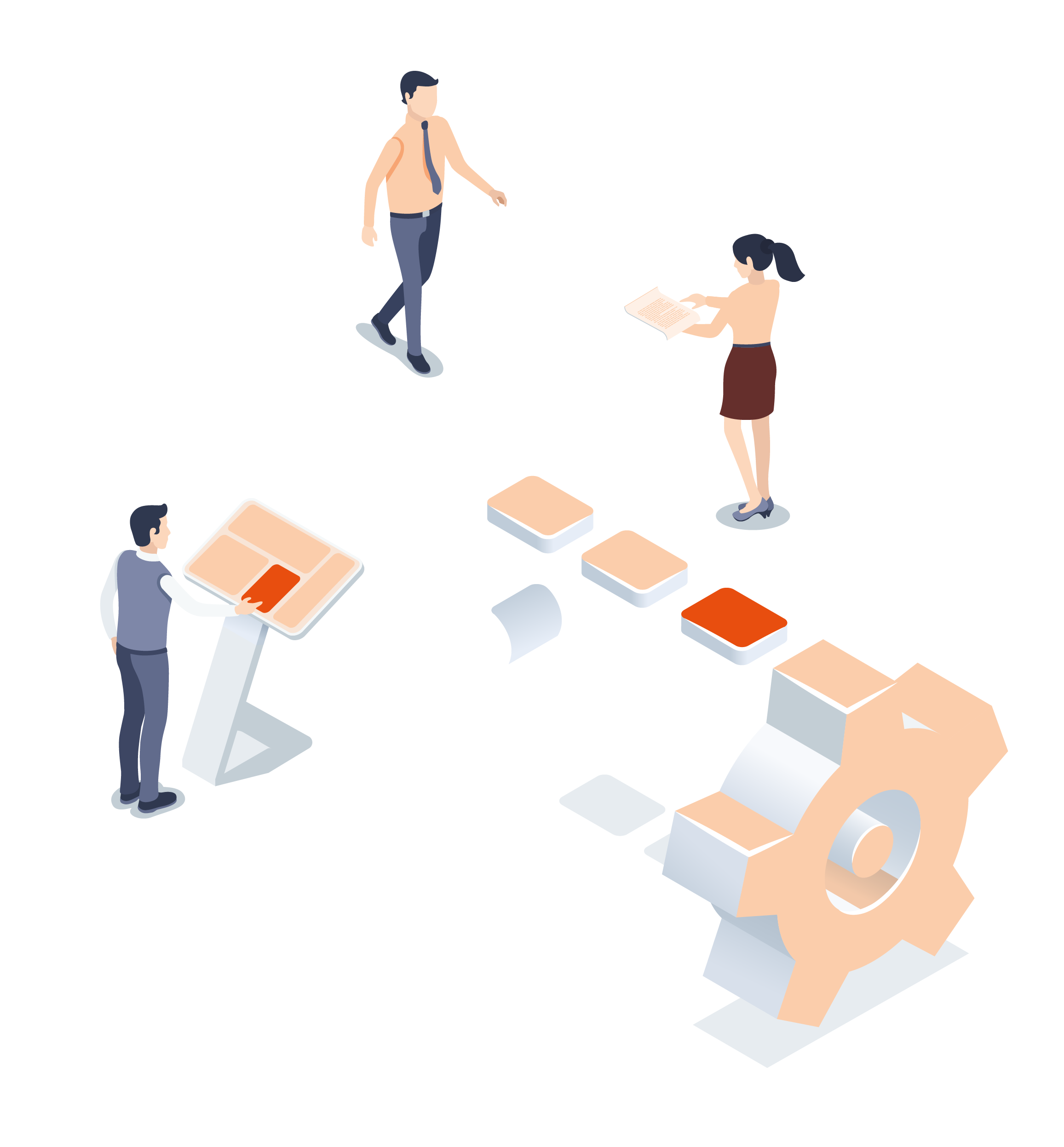
READY FOR THE MARKET
“In the thickener case, our AI solution is tasked with ensuring optimum automatic dosing of the flocculent,” Page says. If the solution proves successful, other customers also stand to benefit in that the algorithm will be offered as a complete package with all the necessary components, such as measuring instruments and edge computers. “At that point, the only thing left to do is adapt the solution to the parameters on the ground. Often, thickening processes may run with different raw materials such as coal, gold or copper, at different temperatures, and with different flocculants,” Page says. “But that’s no longer such a big deal when you’re working closely with the customer concerned.”
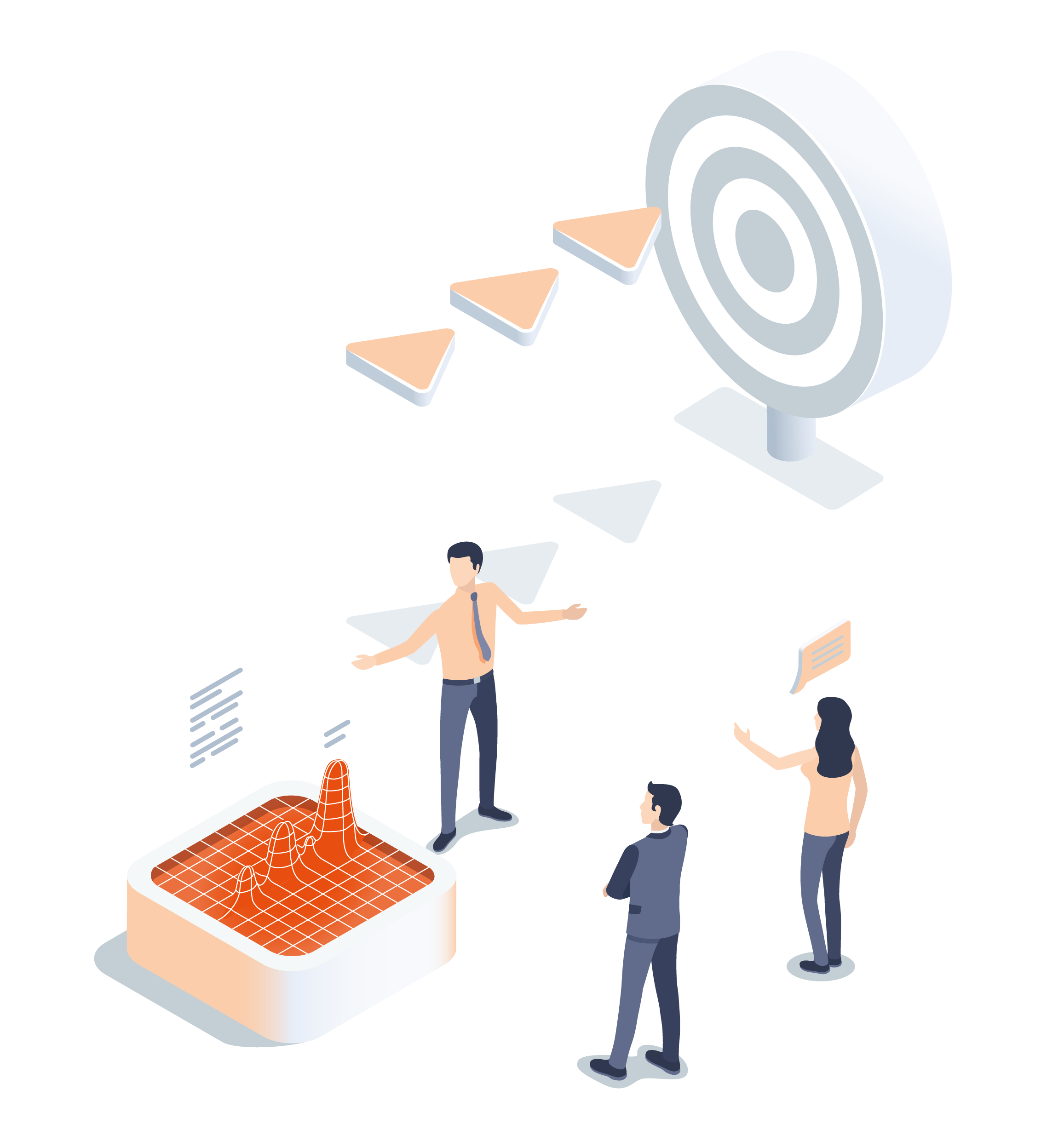
Published 19.09.2024, last updated 21.10.2024.
Dive into the world of the process industry through new exciting stories every month with our «changes» newsletter!
Subscribe to newsletter