Crystal clear
Rapid assessment of measurements at water treatment plants is possible thanks to the experience of the operators. In the future, machine learning could help spot the need for action. Endress+Hauser is working on this kind of assistance system for liquid analysis.
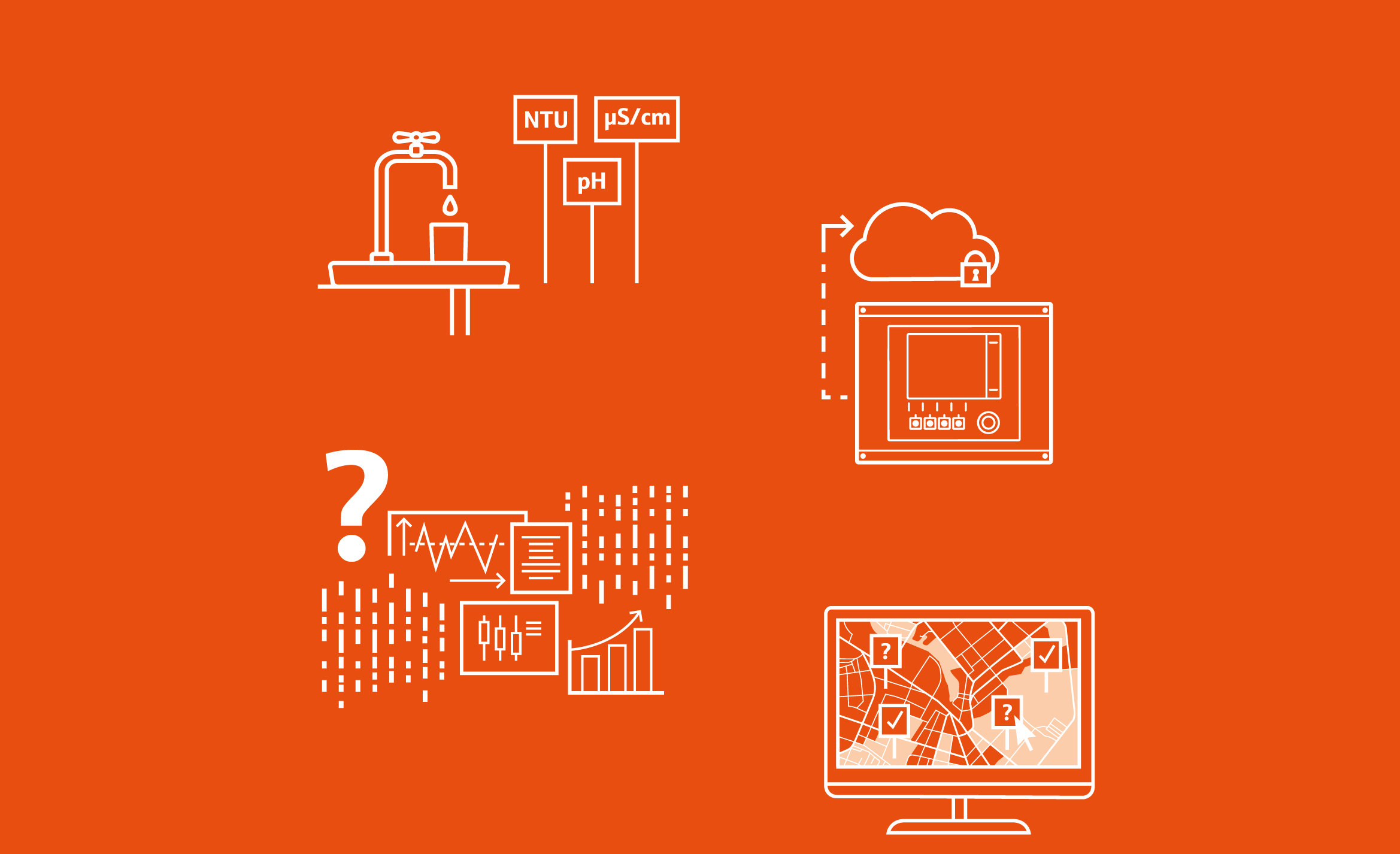
Can I trust the measurement? That’s a question that operating personnel at water treatment plants constantly ask themselves. “The people in the control room must keep a constant eye on all the graphs tracking things like turbidity, pH and conductivity,” says Lars Bondzio, business development manager at the Endress+Hauser product center for liquid analysis. “From experience they can decide whether measurements for a given application are within the usual range.” Outliers or other anomalies could point to problems with the process – or to deposit formation, sensor drift or aging.
“The sheer number of measurements they have to assess can be overwhelming,” says Julia Mildner, head of the service innovation group. Then there is the shortage of skilled and experienced workers, which means that shifts are often understaffed. This is where Endress+Hauser aims to help out with an intelligent assistance system. It uses machine learning algorithms to model the plant operators’ knowledge and automatically check the plausibility of each measurement. To do this, the AI also pulls in historical measurements, diagnostic and verification information from the sensors, and environmental data. “For example, the algorithms can ascertain whether high turbidity levels are the result of heavy rain, meaning everything’s okay, or whether action is required,” Mildner explains.
The new plausibility checks proved a trailblazer in projects undertaken with water suppliers, and are now being developed into a market-ready product called Netilion Liquiline Assist. “Users see tremendous added value in the solution,” says André Lemke, digital portfolio product manager. Netilion Liquiline Assist converts measurement data into easily understood information from which workers can decide on the appropriate response. Inclusion of sensor data could also help to keep measuring points up and running even more reliably: their diagnostic data is noted and trends become apparent. In addition, the plausibility index holds potential as a quality parameter, meaning that comparison measurements in the field are not always necessary.
“The example also illustrates the long innovation timescale for technical changes in the water treatment industry,” says Dr Achim Gahr. As business development manager at Endress+Hauser, he laid the groundwork for automated checking of measurements over 10 years ago. “Now, thanks to digitalization and machine learning, we can finally make such assistance systems a reality.”
Published 22.07.2024, last updated 21.08.2024.
Dive into the world of the process industry through new exciting stories every month with our «changes» newsletter!
Subscribe to newsletter